SARS-CoV-2 Superspreading Events Database
2,000+ Superspreading Events From Around the World
Latest article update: November 21. Latest database update: January 9, 2021.
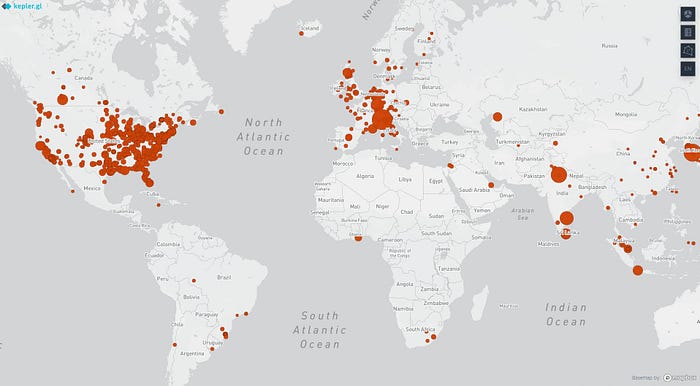
SARS-CoV-2 “superspreading events” (SSEs) occur when a large number of people are infected with SARS-CoV-2 during the same event.
SSEs appear to be the driving force behind the current pandemic. Most infected people don’t infect anybody else, and the ones that do typically only infect one or a few others. But a small number of people infect a lot of others. Multiple studies show that 70–80% of transmissions can be traced back to just 10–20% of cases.
Therefore, in order to have a better chance of containing the virus it is important to investigate the features of SSEs. Specifically, knowing in what types of settings SSEs typically occur may help the public, public health professionals, policy makers, organizations and industry prevent SSEs from happening in the future.
To that end, we have compiled this database with now more than 1,600 SSEs from around the world. For each SSE the following features are identified:
- what the location of the event was
- when the event occurred
- how many people were infected directly and/or indirectly
- what kind of setting the event took place in
- what kind of activity took place
- whether the event took place indoors or outdoors
- whether the event occurred during flu season in that location
The term “events” in this context should be understood in a broad sense, in that the superspreading can take place:
- at the same venue during a short period of time (e.g. at a party)
- at the same venue during an extended period of time (e.g. in a nursing home or prison over the course of several days or weeks)
- at different venues visited by the same superspreader(s)(e.g. an infectious person going on a pub crawl)
Database
Click here to go to the database. Please read the Notes sheet for more information about the database and its limitations.
The database as well as this article will continue to be updated with new SSEs and new information.
The project now also has a website.

Bubble Map with Animated Timeline
Click here to go to the SSE bubble map. Zoom in and click on a bubble to see more information about an SSE.

And click here to see the same map but with an animated timeline that shows the superspreading events as they occurred in time. You can play and pause the animation, and click on a bubble to find more information about an event.

Please note that for most of the large SSEs in the US as well as in some other countries the index dates in the database are placeholders (in the database these cells are marked light red in the Index Date column).
The focus of the database is on the features of the settings in which SSEs took place, and on the general period in which they occurred, specifically whether they occurred in flu season or not. Finding the exact dates for more than 900 SSEs would require a disproportionate amount of time. What this does mean is that no conclusions about the spread of the virus in the US and those countries should be drawn from the timeline.
Preliminary Results
- Nearly all SSEs in the database took place indoors: the exceptions are SSEs that took place in settings with both indoor and outdoor elements and where it is not clear whether transmission occurred indoors or outdoors
- The vast majority of SSE transmissions took place in settings where people were essentially confined together for a prolonged period (for example, nursing homes, prisons, cruise ships, worker housing)
- A feature of these settings is that it is typically outsiders rather than the people who live or work in them (or their relatives) who have control over the circumstances in which they work or live (nursing home residents, hospital patients or inmates typically have little control in terms of precautions they can take)
- The great majority of SSEs happened during flu season in that location
- Food processing plants where temperatures are kept very low (meat, dairy, frozen foods) seem particularly vulnerable to SSEs compared to other types of factories and plants where very few SSEs occurred
These results also point to a theoretical conclusion about the nature of transmission. It seems difficult to explain superspreading events and the fact that superspreading events are vastly more common indoors than outdoors without acknowledging that airborne transmission is key:
- If transmission is primarily through large droplets that don’t travel farther than 6 feet and that fall to the ground almost immediately, we shouldn’t expect superspreading events to be so much more common indoors than outdoors. The behavior of a large droplet is not meaningfully different in an outdoor vs. an indoor setting. It will just fall to the ground almost instantly and not travel farther than 6 feet. Aerosols, on the other hand, are smaller droplets that linger in the air and can travel farther than 6 feet. Aerosols are diluted much faster outdoors than indoors. So the concentration of infectious aerosols in the air people breathe will be much lower outdoors than it is indoors. As a result, superspreading should be much less likely outdoors than indoors. And that is what we see.
- Moreover, at a superspreading event one person (or a small number of people) infects dozens or more others. If transmission happens through large droplets it means that person has to be in close contact with each of those other people and talk, sing, shout, laugh or breathe in their direction. In many situations that seems less likely than that an infected person gradually increases the concentration of infectious aerosols in the air in a poorly ventilated space. The risk will still be highest for people in close contact with the index case because the concentration of infectious aerosols is highest directly in front of the person (similar to standing close to a smoker) but people can become infected at a greater distance as well due to the increased concentration of infectious aerosols in the air they breathe.
Limitations
When assessing these conclusions it is important to keep in mind that the database has some severe limitations. For example, in response to the pandemic societies have taken all sorts of measures to try to contain the virus. This includes the closing of various types of settings , such as restaurants, schools and offices etc. As a result, these settings were then no longer able to give rise to superspreading events. which then were no longer able to give rise to any superspreading events. As a result, certain types of high-risk settings could be significantly underrepresented in the database in the sense that superspreading would have occurred there more frequently if they had been open. To take an obvious example, from the fact that there are no indoor concerts in the database it does not follow that indoor concerts do not pose a serious risk.
Moreover, determining whether an event took place indoors or outdoors (or both) can involve some uncertainty. For categories such as “nursing homes” and “hospitals” we assume that the setting was indoors. But for some other settings such as restaurants or wedding venues we look for more information to make a determination. For example, whenever possible, we:
- look for images of the venue online
- try to find detailed descriptions of the event
- check what the weather was like in that period (eg it is unlikely that a family dinner in Wuhan in January took place outdoors)
This method does not guarantee that the determination is correct 100% of the time. When there is considerable uncertainty about a data point, the corresponding cell in the database is marked light red. We welcome any corrections.
Another limitation of the database involves selection biases that may cause certain types of settings to be overrepresented or underrepresented in the database. For example:
- People may be more likely to remember or mention certain types of settings than other types
- People who are more likely to have been in certain types of settings may be more (or less) easily traceable by contact tracers, or more (or less) willing to be interviewed by them
- In institutional settings such as nursing homes, prisons, hospitals and meat processing plants there will typically be more frequent, systematic & comprehensive testing (especially once one or more people have shown symptoms or tested positive) than in other types of settings such as bars or parties.
There may also be a confirmation bias problem once a specific SSE has been identified. The mere fact that people who now have the virus attended an event that was subsequently identified as an SSE does not mean that those people were infected at that event. Maybe infection occurred elsewhere, before or after. It is by no means always realistically possible to prove with a high degree of confidence when and where transmission occurred. The higher infection rates in a community are, the more of a problem this becomes.
And there is a risk that once an event has been designated as a SSE it develops its own gravitational pull: With the SSE in mind, researchers may more easily assume that newly infected people with a direct or indirect link to the event acquired the infection there — or through somebody who was there — rather than in another way.
In the aggregate this could also lead to an overestimation of the role SSEs play in the pandemic in general.
Furthermore, the lower the incidence of Covid in a population, the more problematic the issue of false positives becomes. False positives can occur in two ways:
- An error during the testing & analysis (including contamination issues in a lab). This is rare.
- The use of high cycle thresholds in PCR testing causes tests to detect insignificant amounts of virus that are not reliable indicators of recent SARS-CoV-2 infections,which may create an inflated impression of the number of current infections in a community, as this New York Times article explains:
Officials at the Wadsworth Center, New York’s state lab, have access to C.T. values from tests they have processed, and analyzed their numbers at The Times’s request. In July, the lab identified 872 positive tests, based on a threshold of 40 cycles.
With a cutoff of 35, about 43 percent of those tests would no longer qualify as positive. About 63 percent would no longer be judged positive if the cycles were limited to 30.
In Massachusetts, from 85 to 90 percent of people who tested positive in July with a cycle threshold of 40 would have been deemed negative if the threshold were 30 cycles, Dr. Mina said. “I would say that none of those people should be contact-traced, not one,” he said.
The higher the rate of false positives, the more the size of superspreading events may be systematically overstated.
Lastly, it should be noted that for settings such as nursing homes and prisons the database typically takes the cumulative number of cases. In reality these infections could have occurred over the course of several days or weeks. Moreover, some people included in this number may have been infected outside of this setting. If the number of infections in a community is significant then the number of staff who were infected outside of this setting may also be significant. The reason these settings are nonetheless included in a database of superspreading events is that due to the nature of these facilities — residents cannot leave and residents typically have been there for a while — the large majority of residents will likely have been infected in the facility itself.
Incomplete and Imperfect
Note that while the goal for the database is to eventually include all SSEs found by researchers and authorities, currently that project is far from completion. Many more SSEs than just these 1,500+ have taken place.
The information in the database is also by no means fully accurate or complete. Dates often had to be guesstimated based on the information available in publications about the events. And, as noted before, placeholders are sometimes used for dates, as well as for the number of cases associated with an SSE. Cells that contain placeholder data or data that needs to be checked for accuracy are marked in light red.
In addition, GPS data for some of the American SSEs may not be accurate as a bulk conversion method was used that may not be 100% accurate.
Also note that for some SSEs only the number of infections at the initial event is included while for others more detailed information about secondary or even tertiary infections was available (via this smaller database, for example) and included in the total number of infections associated with an event. This obviously makes direct quantitative comparisons between SSEs problematic.
Lastly, whenever there are differing estimates of the number of cases associated with an SSE the database always uses the lowest number.
So the database has numerous serious limitations. It is very much imperfect and a work in progress. Please do not assume it is a representative sample of SSEs and please do not draw hasty conclusions from the data.
Feedback and Help
Any help (corrections, additions, suggestions) would be much appreciated. Send your information to info@superspreadingdatabase.com or add them via the Google Form on this page. Assistance with improving the visualization (for example, to make it look more like this) is also welcome.
If you are a researcher who would like to join the project or cooperate in some other way, please send an email to info@superspreadingdatabase.com.
If you'd rather go it alone, the database and all data in it may be freely used by anyone, in whatever way. A link to this article and the database is always appreciated.
How to cite the database
Swinkels, K. (2020). SARS-CoV-2 Superspreading Events Around the World [Google Sheet]. Retrieved from https://docs.google.com/spreadsheets/d/1c9jwMyT1lw2P0d6SDTno6nHLGMtpheO9xJyGHgdBoco/edit?usp=sharing
References
A substantial number of SSEs in this database come from the following sources:
- Quillette database created by Jonathan Kay
- Leclerc et al database (Leclerc, Q.J., Fuller, N.M., Knight, L.E., Funk, S., Knight, G.M. and CMMID COVID-19 Working Group, 2020. What settings have been linked to SARS-CoV-2 transmission clusters?. Wellcome Open Research, 5(83), p.83)
- New York Times article
- Research by Maurice de Hond & Axel van der Kruk
More Articles
- How to Reopen the Economy: 58 Suggestions for Reopening the Economy and Public Life while Continuing to Limit the Damage COVID-19 Is Doing
- 17 Simple Suggestions for Dealing with Future Respiratory Virus Pandemics: Avoid Superspreading Events and you may not need vaccines, contact tracing, lockdowns or any other far-reaching measures
- The Non-Covid Casualties of the Covid Crisis: A Continually Updated Collection of Stories About The Devastating Effects of the Lockdown — (part II, part III)
- Covid-19 Superspreading Events Database: 1,100 Superspreading Events From Around the World
- Covidonomics: What Will the Covid-19 Crisis Do to Our Political Economy?
- Pandemic Threat Inflation: 14 Ways in Which We May Get An Inflated Sense of the Threat Posed by Covid-19